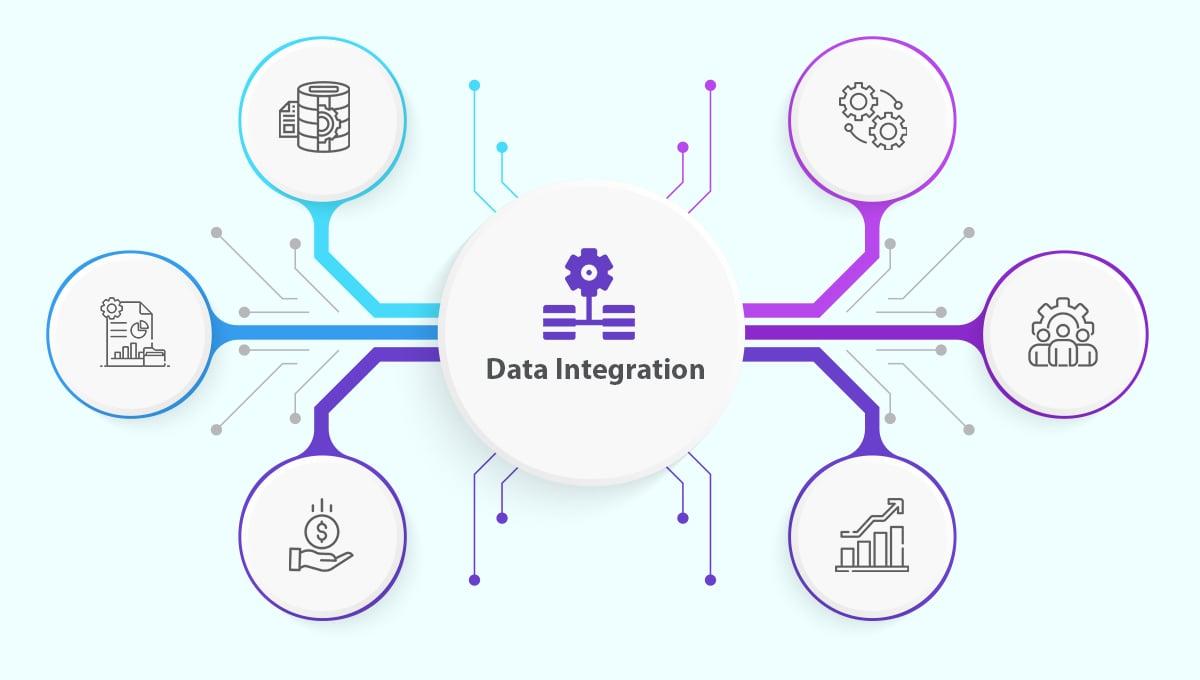
Weaving the Digital Tapestry: A Deep Dive into Data Integration
Introduction:
In the era of big data and digital transformation, organizations grapple with an abundance of information from diverse sources. The challenge lies not only in collecting this data but in making sense of it to drive informed decision-making. Enter data integration—a powerful process that involves combining and unifying disparate datasets to create a cohesive and valuable whole. In this exploration, we delve into the intricacies of data integration, unraveling its importance, methodologies, challenges, and the transformative impact it holds for businesses and beyond. Click here to read more content.
Defining Data Integration:
At its core, data integration is the process of combining data from different sources to provide a unified view. This amalgamation involves harmonizing diverse data formats, structures, and systems, enabling seamless interaction and analysis. The goal is to create a cohesive, accurate, and comprehensive dataset that empowers organizations with a holistic understanding of their operations.
The Imperative of Unified Data:
In a business landscape inundated with information silos, achieving a unified view of data is imperative. Data integration transcends the boundaries between departments, systems, and formats, fostering a cohesive environment where insights can be derived from a consolidated, accurate, and up-to-date dataset. This unified data is the foundation for strategic decision-making and innovation.
Methods of Data Integration:
Data integration involves a variety of methods, each tailored to the specific needs and complexities of the data landscape. These methods include:
Batch Processing:
Involves collecting and processing data in predefined batches, usually during off-peak hours.
Real-time Integration:
Enables data to be processed and updated in real-time, providing immediate insights and reactions to changing conditions.
Middleware Integration:
Utilizes middleware software to facilitate communication and data exchange between disparate systems.
Data Warehousing:
Involves storing and consolidating data from multiple sources in a central repository for analytical purposes.
Application-Based Integration:
Integrates data at the application level, ensuring that different software applications can communicate seamlessly.
Key Components of Data Integration:
Extraction, Transformation, Load (ETL):
ETL processes form the backbone of data integration. Data is extracted from source systems, transformed to meet the desired format, and loaded into the target system. This ensures data consistency and compatibility.
Data Mapping:
Involves creating a relationship between data elements in different systems, ensuring accurate alignment during integration.
Data Quality Management:
Ensures the integrity of integrated data by identifying and rectifying errors, duplications, and inconsistencies.
Metadata Management:
Governs the documentation and understanding of data, providing crucial context for its interpretation and application.
Data Governance:
Establishes policies, processes, and standards to ensure the ethical and secure use of integrated data.
Benefits of Data Integration:
Enhanced Decision-Making:
A unified view of data empowers organizations to make more informed, strategic decisions based on a comprehensive understanding of their operations.
Improved Operational Efficiency:
Data integration streamlines processes by eliminating redundancies, reducing errors, and enhancing workflow efficiency.
Customer Insights:
By integrating customer data from various touchpoints, businesses gain a holistic view of customer behavior, enabling personalized and targeted approaches.
Innovation Acceleration:
Access to consolidated data fuels innovation by providing a fertile ground for advanced analytics, machine learning, and artificial intelligence applications.
Cost Savings:
Eliminating duplicate efforts, reducing errors, and optimizing resource allocation contribute to significant cost savings.
Regulatory Compliance:
Data integration facilitates adherence to regulatory standards by ensuring accurate reporting and data management practices.
Challenges in Data Integration:
Data Quality:
Ensuring the quality of integrated data is a persistent challenge. Inaccuracies, inconsistencies, and errors in the source data can propagate into the integrated dataset.
Data Security:
The integration of data from multiple sources raises concerns about data security and privacy. Safeguarding sensitive information becomes paramount.
Compatibility Issues:
Different data formats, structures, and systems may pose compatibility challenges during the integration process.
Scalability:
As data volumes grow, ensuring the scalability of data integration processes becomes crucial to maintain efficiency and performance.
Change Management:
Integrating new data sources or modifying existing ones requires careful change management to prevent disruptions and ensure a smooth transition.
Resource Intensiveness:
The implementation and maintenance of data integration processes may demand significant resources, both in terms of technology and skilled personnel.
Emerging Trends in Data Integration:
Cloud-Based Integration:
Leveraging cloud platforms for data integration offers scalability, flexibility, and cost-effectiveness, enabling organizations to adapt to dynamic data landscapes.
Data Mesh:
This innovative approach decentralizes data ownership and processing, treating data as a product and fostering a more scalable and agile data ecosystem.
AI and Machine Learning Integration:
Integrating artificial intelligence and machine learning into data integration processes enhances automation, anomaly detection, and predictive analytics.
Self-Service Data Integration:
Empowering non-technical users with tools for self-service data integration reduces dependence on IT departments and accelerates decision-making.
Blockchain Integration:
Blockchain technology ensures the immutability and security of integrated data, particularly valuable in industries with high regulatory requirements.
Applications Across Industries:
Healthcare:
Data integration facilitates a unified patient record, improving care coordination, and enhancing healthcare outcomes.
Retail:
Integrated data enables retailers to gain insights into customer behavior, optimize supply chains, and implement personalized marketing strategies.
Finance:
In the financial sector, data integration is crucial for risk management, fraud detection, and compliance with regulatory standards.
Manufacturing:
Integrating data from production processes, supply chains, and quality control systems enhances efficiency and enables predictive maintenance.
Telecommunications:
Data integration is essential in the telecommunications industry for managing network performance, customer billing, and improving service quality.
The Road Ahead:
As the digital landscape continues to evolve, the importance of data integration will only intensify. Organizations must embrace innovative technologies, adhere to best practices, and foster a culture of data-driven decision-making to successfully navigate the complexities of the modern world. Data integration is not merely a technical process; it is a strategic imperative that empowers organizations to harness the full potential of their data for a more agile, informed, and resilient future.
Conclusion: Stitching the Future of Insights:
In an era where data is likened to the new oil, data integration emerges as the refining process that transforms raw information into actionable insights. The complexities of the modern world demand a sophisticated approach to managing and utilizing data effectively. By embracing data integration as a strategic initiative, organizations can stitch together a future where data becomes a valuable asset, driving innovation, fostering informed decision-making, and ultimately propelling them ahead in the digital age.